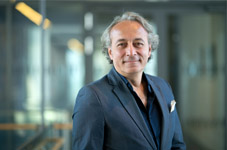
Lehrgebiet: Theoretische Informatik und künstliche Intelligenz
Büro: 01.214
Labor: 04.105
Telefon: +49 208 88254-806
E-Mail:
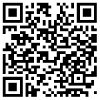
Ioannis Iossifidis studierte Physik (Schwerpunkt: theoretische Teilchenphysik) an der Universität Dortmund und promovierte 2006 an der Fakultät für Physik und Astronomie der Ruhr-Universität Bochum.
Am Institut für Neuroinformatik leitete Prof. Dr. Iossifidis die Arbeitsgruppe Autonome Robotik und nahm mit seiner Forschungsgruppe erfolgreich an zahlreichen, vom BmBF und der EU, geförderten Forschungsprojekten aus dem Bereich der künstlichen Intelligenz teil. Seit dem 1. Oktober 2010 arbeitet er an der HRW am Institut Informatik und hält den Lehrstuhl für Theoretische Informatik – Künstliche Intelligenz.
Prof. Dr. Ioannis Iossifidis entwickelt seit über 20 Jahren biologisch inspirierte anthropomorphe, autonome Robotersysteme, die zugleich Teil und Ergebnis seiner Forschung im Bereich der rechnergestützten Neurowissenschaften sind. In diesem Rahmen entwickelte er Modelle zur Informationsverarbeitung im menschlichen Gehirn und wendete diese auf technische Systeme an.
Ausgewiesene Schwerpunkte seiner wissenschaftlichen Arbeit der letzten Jahre sind die Modellierung menschlicher Armbewegungen, der Entwurf von sogenannten «Simulierten Realitäten» zur Simulation und Evaluation der Interaktionen zwischen Mensch, Maschine und Umwelt sowie die Entwicklung von kortikalen exoprothetischen Komponenten. Entwicklung der Theorie und Anwendung von Algorithmen des maschinellen Lernens auf Basis tiefer neuronaler Architekturen bilden das Querschnittsthema seiner Forschung.
Ioannis Iossifidis’ Forschung wurde u.a. mit Fördermitteln im Rahmen großer Förderprojekte des BmBF (NEUROS, MORPHA, LOKI, DESIRE, Bernstein Fokus: Neuronale Grundlagen des Lernens etc.), der DFG («Motor‐parietal cortical neuroprosthesis with somatosensory feedback for restoring hand and arm functions in tetraplegic patients») und der EU (Neural Dynamics – EU (STREP), EUCogII, EUCogIII ) honoriert und gehört zu den Gewinnern der Leitmarktwettbewerbe Gesundheit.NRW und IKT.NRW 2019.
ARBEITS- UND FORSCHUNGSSCHWERPUNKTE
- Computational Neuroscience
- Brain Computer Interfaces
- Entwicklung kortikaler exoprothetischer Komponenten
- Theorie neuronaler Netze
- Modellierung menschlicher Armbewegungen
- Simulierte Realität
WISSENSCHAFTLICHE EINRICHTUNGEN
- Labor mit Verlinkung
- ???
- ???
LEHRVERANSTALTUNGEN
- ???
- ???
- ???
PROJEKTE
- Projekt mit Verlinkung
- ???
- ???
WISSENSCHAFTLICHE MITARBEITER*INNEN
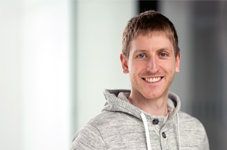
Felix Grün
Büro: 02.216 (Campus Bottrop)
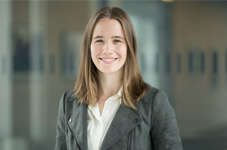
Marie Schmidt
Büro: 02.216 (Campus Bottrop)
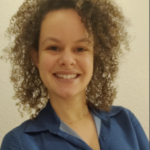
Aline Xavier Fidencio
Gastwissenschaftlerin
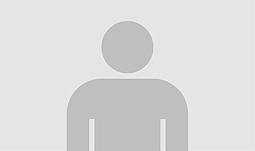
Muhammad Ayaz Hussain
Doktorand
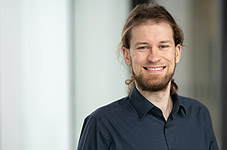
Tim Sziburis
Doktorand
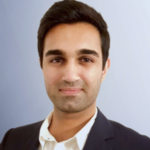
Farhad Rahmat
studentische Hilfskraft
AUSGEWÄHLTE PUBLIKATIONEN
-
Es sind leider keine Einträge vorhanden.
RESEARCHGATE PROFIL
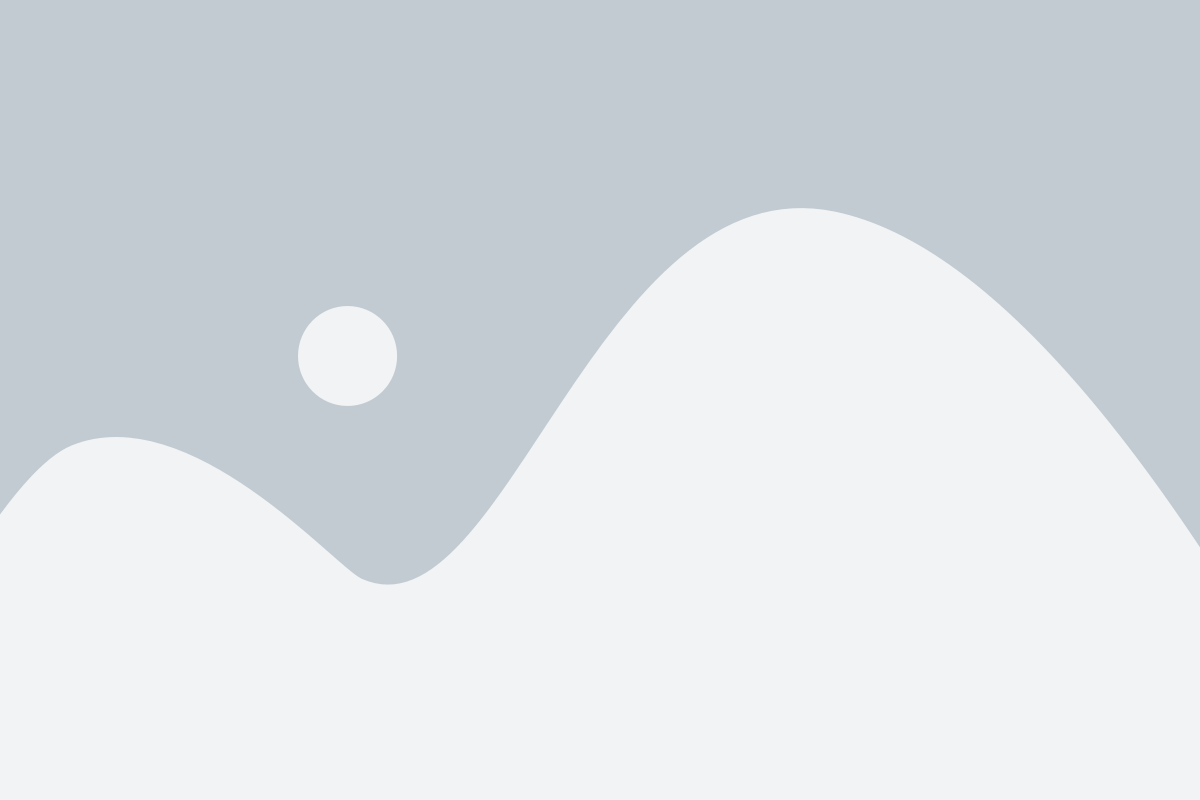
GOOGLE SCHOLAR PROFIL
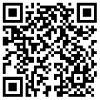